Key Takeaways:
I. Silicon's inherent limitations are hindering the progress of AI, necessitating the exploration of alternative materials.
II. Novel materials like GaN, SiC, graphene, and carbon nanotubes offer superior properties but present integration and manufacturing challenges.
III. Advanced architectures like 3D chip stacking and heterogeneous integration are crucial for maximizing the potential of new materials and shaping the future of AI hardware.
The rapid advancement of artificial intelligence (AI), particularly with the rise of large language models and deep learning, is placing unprecedented demands on computing hardware. While Moore's Law has driven decades of progress in semiconductor technology, we are approaching the physical limits of silicon. Current AI systems require exponentially increasing computational power, memory bandwidth, and energy efficiency, pushing silicon-based technology to its breaking point. To sustain the momentum of AI innovation, a fundamental shift is required: a materials science revolution that explores and integrates novel materials with superior properties.
The End of Moore's Law: Rethinking AI Hardware
Silicon's electron mobility, a crucial determinant of transistor speed, is inherently limited. At approximately 1400 cm²/Vs, silicon's electron mobility pales in comparison to materials like graphene, which boasts mobilities exceeding 200,000 cm²/Vs. This difference translates to significantly slower switching speeds in silicon transistors, hindering the performance of AI algorithms that demand rapid data processing.
Silicon's thermal conductivity, a measure of its ability to dissipate heat, is another critical limitation. With a thermal conductivity of around 150 W/mK, silicon struggles to manage the heat generated by power-hungry AI computations. This can lead to overheating, performance degradation, and increased energy consumption. Materials like SiC (490 W/mK) and diamond (2200 W/mK) offer superior thermal management capabilities, enabling higher power densities and improved efficiency.
The continued miniaturization of silicon transistors, a key driver of Moore's Law, is facing fundamental physical limits. As transistors approach the atomic scale, quantum effects like tunneling become significant, leading to increased leakage current and reduced performance. These challenges make it increasingly difficult and expensive to pack more transistors onto a chip, hindering further performance gains.
These limitations in electron mobility, thermal conductivity, and scalability collectively create a significant bottleneck for AI development. As AI algorithms become more complex and data sets grow larger, the demands on computing hardware continue to escalate, exposing the shortcomings of silicon and necessitating the exploration of alternative materials and architectures.
Beyond Silicon: The Search for AI's Material Foundation
Wide bandgap semiconductors, such as Gallium Nitride (GaN) and Silicon Carbide (SiC), offer a compelling near-term alternative to silicon. Their higher electron mobility and superior thermal conductivity enable faster switching speeds and greater power handling capabilities, making them well-suited for high-performance AI applications. However, integrating these materials into existing silicon-based fabrication processes presents challenges related to material compatibility and defect management.
Two-dimensional (2D) materials, including graphene and transition metal dichalcogenides (TMDs), represent a more radical departure from traditional semiconductors. Graphene, with its exceptional electron mobility and atomic thinness, holds the potential for ultra-fast and energy-efficient transistors. TMDs offer tunable electronic properties, making them suitable for a variety of AI applications. However, challenges in large-scale, defect-free production and integration with existing CMOS technology remain significant hurdles.
Carbon nanotubes (CNTs), cylindrical structures composed of rolled-up graphene sheets, possess remarkable electrical and thermal properties. Their high electron mobility and thermal conductivity could enable the development of ultra-fast and energy-efficient devices. However, challenges in controlled synthesis, alignment, and integration into functional circuits limit their current applicability in AI hardware.
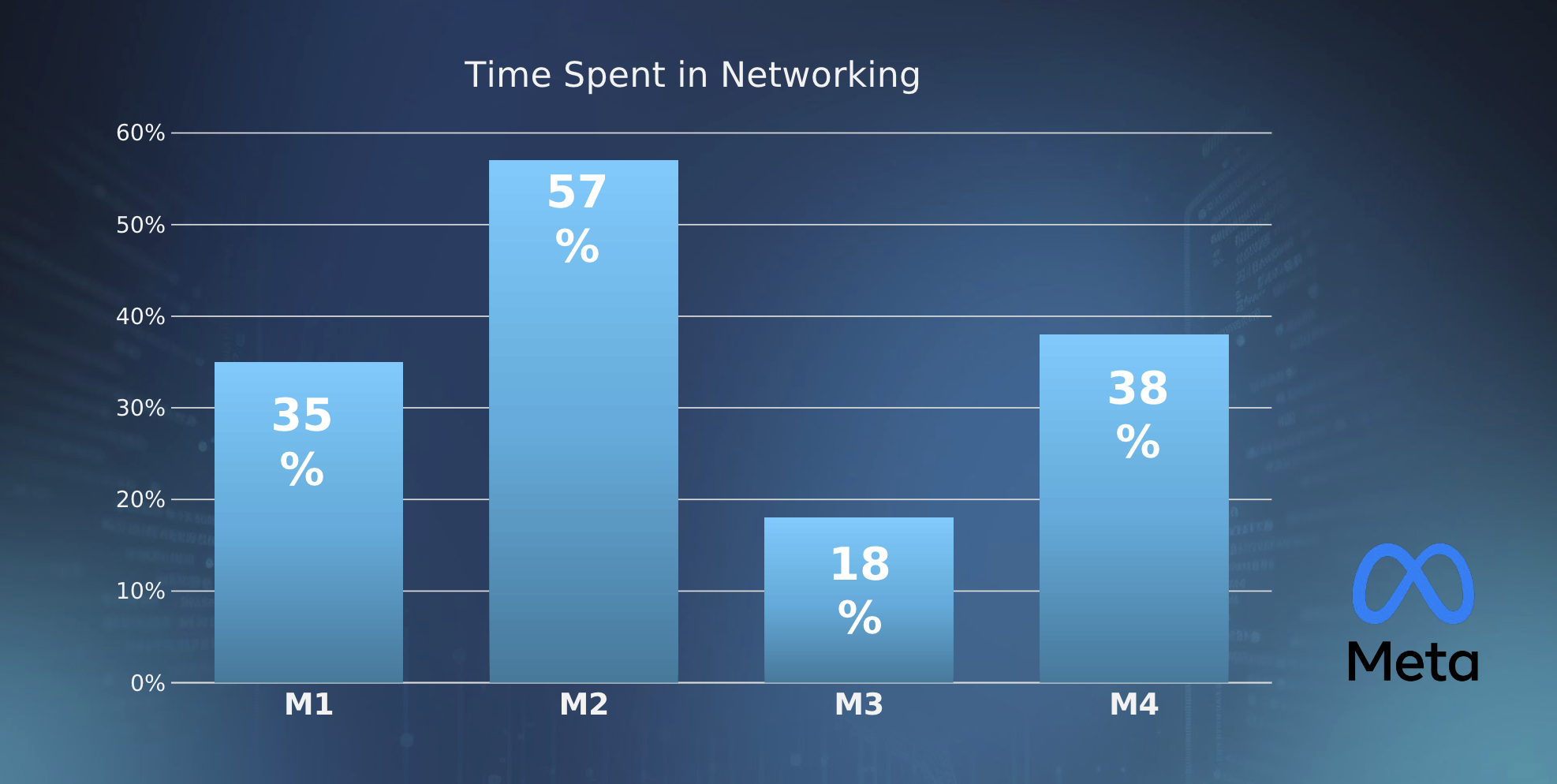
The successful implementation of any of these alternative materials requires a holistic approach that considers not only their intrinsic properties but also their manufacturability, cost-effectiveness, and compatibility with existing infrastructure. The transition beyond silicon will require significant investments in research, development, and infrastructure, as well as close collaboration between academia, industry, and government.
Beyond Planar Designs: New Architectures for the Age of AI
Three-dimensional (3D) chip stacking, by vertically integrating multiple chip layers, offers a powerful approach to increase memory bandwidth, reduce interconnect lengths, and improve overall system performance. However, this architecture presents significant thermal management challenges, requiring advanced cooling solutions and materials with high thermal conductivity to prevent overheating and ensure reliable operation.
Heterogeneous integration, which combines different materials and device types on a single chip, allows for customized optimization of AI hardware. By integrating specialized processing units like GPUs and ASICs with memory and other components, heterogeneous integration can significantly enhance performance and efficiency. However, this approach requires advanced packaging techniques and careful interface engineering to ensure seamless communication and compatibility between different elements.
The Future of AI Hardware: A Collaborative Roadmap
The path forward for AI hardware lies in a collaborative and interdisciplinary approach. Materials scientists, chemists, physicists, and computer scientists must work together to overcome the limitations of silicon and unlock the full potential of AI. This requires not only continued investment in research and development but also a focus on scalable manufacturing processes, cost-effective solutions, and a sustainable approach to materials selection and usage. The future of AI is not just about building faster chips; it's about building a future where AI can address some of humanity's most pressing challenges.
----------
Further Reads
I. https://securityintelligence.com/articles/trends-hardware-gets-ai-updates-2024/Trends: Hardware gets AI updates in 2024
II. https://www.hpcwire.com/2024/04/24/intels-silicon-brain-system-a-blueprint-for-future-ai-computing-architectures/Intel's Silicon Brain System a Blueprint for Future AI Computing Architectures
III. https://www.mckinsey.com/~/media/McKinsey/Industries/Semiconductors/Our Insights/Artificial intelligence hardware New opportunities for semiconductor companies/Artificial-intelligence-hardware.ashxArtificial-intelligence hardware: New opportunities for ...