Key Takeaways:
I. Shutterstock's research license significantly lowers the barrier to entry for AI research, potentially fostering a wave of innovation from startups and smaller teams.
II. The initiative's commitment to ethical sourcing and creator compensation sets a positive precedent, but greater transparency is needed to ensure accountability.
III. Navigating the complex and evolving legal landscape surrounding AI training data requires continuous adaptation and proactive engagement with emerging challenges.
In a move poised to reshape the landscape of artificial intelligence development, Shutterstock has partnered with Lightricks to introduce a novel 'research license' for AI training data. This initiative directly addresses a critical bottleneck in the field: the often prohibitive cost and complexity of acquiring high-quality training data, particularly for startups and smaller research teams. By granting access to its vast library of HD and 4K video footage, Shutterstock aims to democratize AI research and development, fostering innovation and accelerating the development of cutting-edge AI models. This partnership also seeks to establish a more ethical and legally sound framework for data acquisition, promising fair compensation for creators and navigating the complex legal challenges surrounding AI training data. This article delves into the technical, ethical, and legal implications of Shutterstock's research license, offering a comprehensive analysis for industry stakeholders, engineers, and policymakers.
Unlocking AI's Potential: A Deep Dive into Shutterstock's Data Offering
The quality of training data is paramount in shaping the performance, accuracy, and bias of AI models. High-resolution images and videos allow for the capture of fine-grained details, enabling models to make more nuanced predictions. Diversity in the dataset, encompassing a wide range of scenarios, demographics, and visual styles, is crucial for mitigating bias and ensuring that AI systems perform equitably across different contexts. Furthermore, accurate and comprehensive metadata facilitates efficient data retrieval, organization, and analysis, streamlining the training process and empowering researchers to extract valuable insights.
Shutterstock's data offering stands out for its sheer scale and resolution. With over one billion royalty-free assets, including a vast library of HD and 4K videos, the platform provides a rich resource for training data-hungry AI models. The company's emphasis on 'meticulously reviewed' metadata further enhances the value proposition, promising researchers access to well-organized and accurately labeled data. This comprehensive approach to data curation positions Shutterstock as a potential leader in the rapidly evolving AI training data market.
However, a closer examination reveals a need for greater transparency regarding the specifics of Shutterstock's dataset. While the platform emphasizes quality and diversity, it lacks detailed information about the composition of its library. Specific metrics regarding the representation of various demographics, cultures, and visual styles are crucial for researchers to assess potential biases and ensure that their AI models are trained on truly representative data. This lack of transparency hinders a comprehensive evaluation of the dataset's suitability for training unbiased and equitable AI systems.
Furthermore, more detailed technical specifications are needed to fully understand the capabilities and limitations of Shutterstock's data offering. Information regarding frame rates, codecs used, the depth and structure of metadata annotations, and the availability of specialized datasets for specific AI tasks would empower researchers to make informed decisions. Greater transparency in these technical aspects is essential for fostering trust and enabling researchers to fully leverage the platform's potential for advancing AI development.
Creator Compensation and Consent: Navigating the Ethical Landscape of AI Training Data
The use of data to train AI models raises complex ethical considerations that must be addressed proactively. One of the most pressing issues is creator compensation. How are the creators of the images and videos used for training fairly compensated for their work? Shutterstock's revenue-sharing model, offering creators 20% of the proceeds from data licensing deals, is a step in the right direction. However, greater transparency is needed regarding how this revenue is calculated and distributed to ensure equitable compensation.
Equally important is the issue of informed consent. Creators must be fully aware of how their data will be used and have the option to opt out if they choose. While Shutterstock provides an opt-out mechanism, the specifics of how creators are informed about data usage and their opt-out rights require greater clarity. Transparent and readily accessible information about data usage and consent procedures is crucial for building trust and fostering a responsible AI data ecosystem.
Another critical ethical challenge is the potential for bias in training data. Even with a vast and diverse library like Shutterstock's, biases can still emerge if certain demographics, cultures, or perspectives are underrepresented. Proactive measures are needed to identify and mitigate potential biases, including careful curation of the dataset, data augmentation techniques to address imbalances, and ongoing monitoring and evaluation to ensure that biases are not introduced or amplified over time.
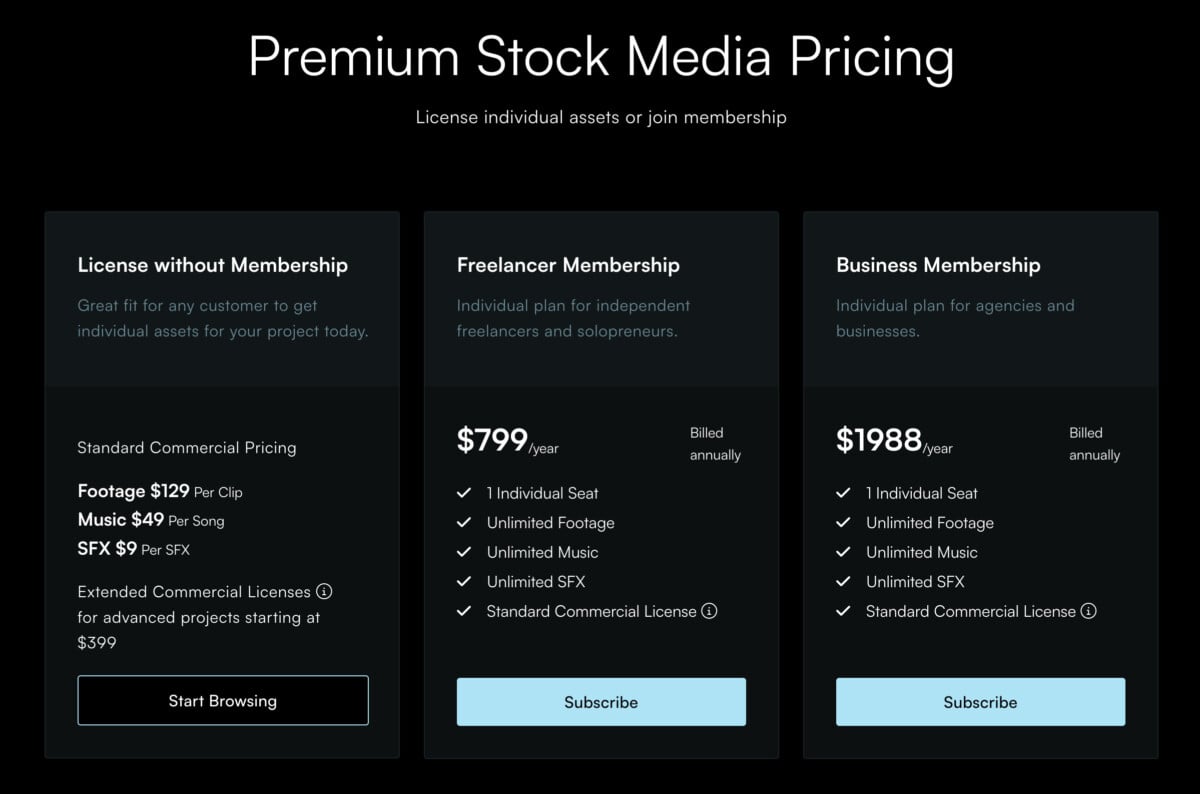
The ethical implications of AI training data extend beyond the immediate creators and users of the data. The AI models trained on this data will be deployed in various applications, potentially impacting individuals and communities in significant ways. Therefore, a comprehensive ethical framework must consider the broader societal implications of these models, addressing questions of fairness, accountability, transparency, and the potential for discriminatory outcomes.
Legal and Regulatory Considerations for AI Training Data
The legal and regulatory landscape surrounding AI training data is complex and rapidly evolving. Data privacy regulations like GDPR and CCPA impose strict requirements on data collection, storage, and usage, impacting how AI training data can be sourced and utilized. Intellectual property rights, including copyright and fair use, are central to the debate surrounding the use of copyrighted material for AI training. The California AI Transparency Act, enacted in September 2024, adds another layer of complexity by mandating disclosures regarding training data for AI systems. Navigating these legal and regulatory challenges is crucial for ensuring the responsible and sustainable development of AI.
Shutterstock's research license aims to provide a legally sound framework for accessing and utilizing training data. However, greater transparency is needed regarding the specific mechanisms for ensuring compliance with GDPR, CCPA, and the California AI Transparency Act. How does Shutterstock address data ownership rights and intellectual property considerations? What are its procedures for obtaining and documenting creator consent? Clear and readily accessible information about these legal and compliance aspects is essential for building trust and fostering a responsible AI data ecosystem.
Shaping the Future of AI: A Call for Transparency, Accountability, and Collaboration
Shutterstock's research license represents a significant development in the quest to democratize AI. By lowering the barriers to accessing high-quality training data, it has the potential to unlock a wave of innovation from startups and smaller research teams. However, realizing this potential requires a commitment to transparency, accountability, and ethical data practices. Clearer communication about creator compensation, robust consent procedures, and proactive bias mitigation strategies are essential for building trust and fostering a responsible AI ecosystem. Furthermore, ongoing engagement with the evolving legal and regulatory landscape is crucial for ensuring the long-term sustainability of this initiative. The future of AI depends on collaborative efforts between industry stakeholders, policymakers, and researchers to navigate the complex challenges and opportunities of this transformative technology.
----------
Further Reads
I. What does Shutterstock data license mean? - Edwin Muller Photography
II. Data licensing from Shutterstock | Train your AI models with the best multimodal data
III. Shutterstock made AI-training deals with everyone, apparently. - The Verge