Key Takeaways:
I. AI is revolutionizing biologics drug discovery, offering significant potential for cost reduction, accelerated timelines, and improved success rates.
II. The surge in M&A activity, funding, and partnerships in the AI drug discovery space underscores the growing confidence in AI's ability to reshape the future of medicine.
III. Addressing ethical considerations, developing robust regulatory frameworks, and prioritizing data quality are crucial for realizing the full potential of AI in biologics drug discovery.
Bringing a new drug to market is a notoriously expensive and time-consuming endeavor. Traditional drug development involves staggering costs, averaging $1.3 billion when accounting for all failed compounds, and timelines often exceeding a decade. This high failure rate, coupled with increasing R&D costs, poses a significant challenge to the pharmaceutical industry. Artificial intelligence (AI) has emerged as a potential solution, promising to accelerate drug discovery, reduce costs, and improve the probability of success, particularly in the rapidly evolving field of biologics. This article explores the transformative impact of AI on biologics drug discovery, analyzing the technical advancements, market dynamics, and ethical considerations shaping this burgeoning field.
AI is demonstrating remarkable capabilities in several key areas of biologics drug discovery. In molecular docking, AI algorithms, exemplified by DeepMind's AlphaFold2, are significantly enhancing the prediction of ligand-protein binding affinities, surpassing traditional methods in both speed and accuracy. This allows researchers to explore potential binding sites with greater precision and efficiency, reducing the reliance on time-consuming and expensive experimental techniques. AI is also revolutionizing virtual screening, enabling the rapid identification of potential drug candidates from vast chemical libraries. Machine learning algorithms can analyze massive datasets of molecular properties and biological activities to pinpoint promising compounds with a higher likelihood of success, streamlining the early stages of drug discovery.
Despite these advancements, current AI algorithms face limitations. The accuracy of docking predictions is still influenced by the quality of the protein structure and the force fields used in the simulations. Furthermore, the complexity of allosteric interactions and dynamic conformational changes in proteins poses challenges for current AI models. In virtual screening, the effectiveness is heavily dependent on the quality and size of the training datasets. Bias in the data can lead to skewed predictions, and the lack of sufficient data for certain target classes can limit the applicability of AI-based methods. Predicting off-target effects and potential toxicities remains a significant hurdle, requiring further development of sophisticated AI models capable of integrating diverse data sources and predicting complex biological interactions.
Note: This visualization represents a snapshot of 2024 deal activity. Further analysis is needed to understand the underlying market dynamics and specific therapeutic area trends.
Predictive modeling of drug efficacy and safety is another area where AI is showing promise, but challenges remain. Machine learning models can integrate diverse data types, including genomic, proteomic, and clinical data, to predict a drug's effectiveness and potential side effects. However, the complexity of human biology and the multitude of factors influencing drug response pose significant challenges for predictive modeling. The accuracy of predictions is often limited by the availability of high-quality, comprehensive data, and the inherent variability in human responses to drugs. Furthermore, the 'black box' nature of some AI models can make it difficult to interpret their predictions and understand the underlying mechanisms, limiting their acceptance within the regulatory environment.
The computational requirements for AI-driven drug discovery, particularly for biologics, are substantial. Training deep learning models on large datasets of protein sequences, structures, and biological activities requires significant computing power and specialized hardware, such as GPUs and TPUs. This can be a barrier to entry for smaller research groups and companies with limited resources. Furthermore, the development of novel AI algorithms specifically designed for handling the unique complexities of biologics, such as their conformational flexibility and post-translational modifications, requires ongoing research and development efforts. The need for high-performance computing infrastructure and specialized expertise in both AI and molecular biology represents a significant challenge for widespread adoption.
The AI drug discovery market is experiencing rapid growth, driven by increased M&A activity and a surge in funding for biologics. Eight of the ten largest deals in the sector have occurred since 2023, reflecting a maturing technology and growing urgency among larger players to bring AI tech in-house. Biologics developers using AI have secured $1.6 billion in funding in 2024, more than double the 2023 amount, with Xaira Therapeutics’ $1 billion raise leading the way. This focus on biologics signals that AI capabilities are broadening from small molecules to larger and more complex therapeutics.
All ten of the largest big pharma players globally have partnered with AI drug discovery startups since 2023, while nine out of ten are simultaneously developing in-house AI capabilities. They are also acquiring therapeutics from AI drug discovery players, as seen with Takeda's $4 billion acquisition of Nimbus Therapeutics’ plaque psoriasis treatment in 2023. These strategic partnerships and acquisitions highlight the growing importance of AI in drug development and the increasing integration of AI technologies into the pharmaceutical industry.
The strategic implications of AI in drug discovery are profound, impacting R&D investment, competitive dynamics, and the formation of new partnerships. Pharmaceutical companies are increasingly recognizing the potential of AI to accelerate drug development and reduce costs, leading to significant investments in AI-related technologies and expertise. This includes both internal development of AI capabilities and collaborations with external AI companies. The increased investment in AI is reshaping the competitive landscape of the pharmaceutical industry, with companies that effectively leverage AI gaining a significant advantage in terms of speed, efficiency, and innovation.
The shift towards AI-driven drug discovery is also influencing the types of therapeutics being developed. While small molecule drugs have traditionally been the focus, AI is enabling the development of more complex biologics, including antibodies, cell therapies, and gene therapies. These novel therapeutic modalities offer the potential to treat a wider range of diseases with greater precision and efficacy, but they also present unique challenges in terms of development, manufacturing, and regulation.
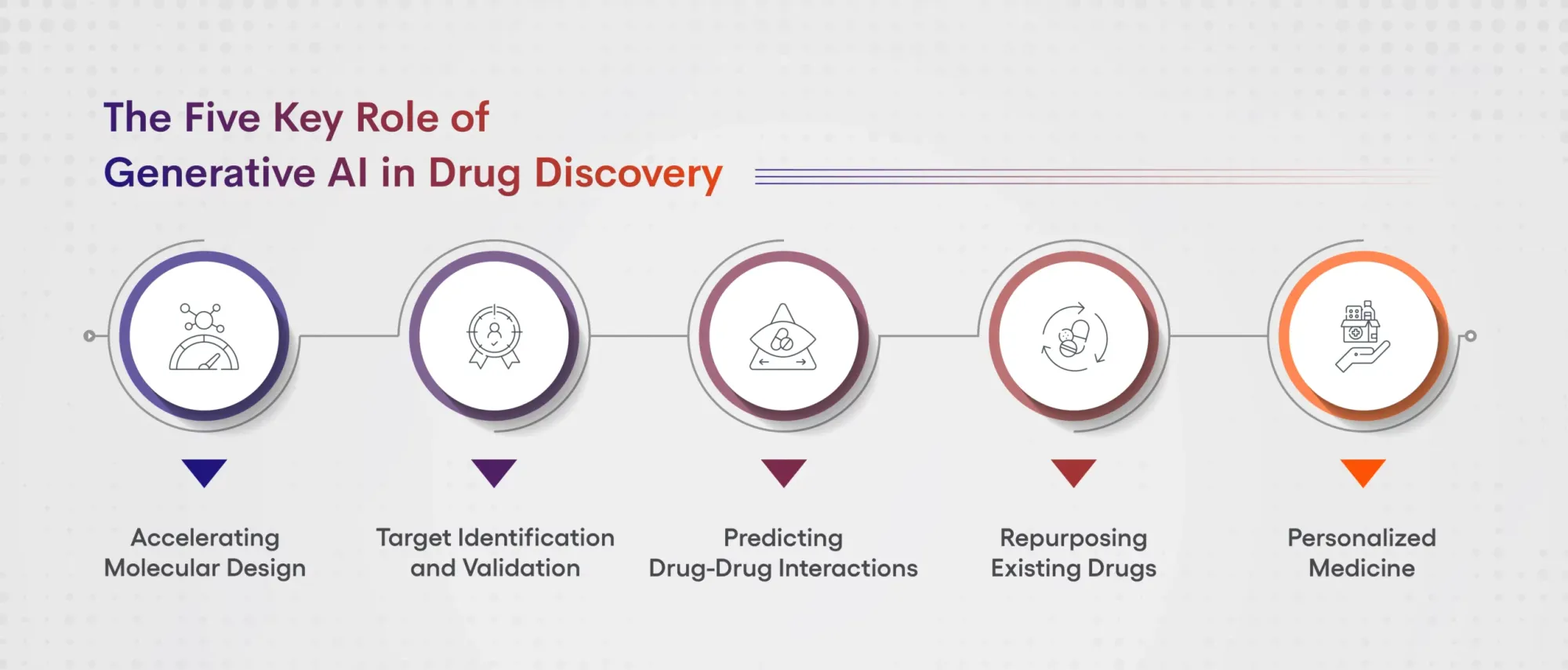
The deployment of AI in healthcare raises important ethical considerations, particularly regarding data privacy. AI algorithms require access to vast amounts of patient data, raising concerns about the security and confidentiality of sensitive health information. Robust data anonymization and encryption techniques are crucial to mitigate these risks, and stringent regulatory frameworks are necessary to ensure compliance with privacy regulations such as HIPAA and GDPR. Federated learning techniques, which allow AI models to be trained on decentralized datasets without directly accessing the raw data, offer a promising approach to address data privacy concerns while enabling collaborative research.
Algorithmic bias is another major concern. AI models trained on biased data can perpetuate and even amplify existing biases in healthcare, leading to disparities in access and quality of care. Careful curation of training datasets, rigorous testing for bias, and ongoing monitoring of AI algorithms are crucial to mitigate the risk of algorithmic bias. Furthermore, the need for regulatory frameworks that can keep pace with technological advancements is critical. The rapid evolution of AI necessitates the development of flexible and adaptable regulatory pathways for AI-based medical devices and diagnostic tools. Collaboration between regulatory bodies, AI developers, and healthcare professionals is essential to create effective and responsible regulatory frameworks that foster innovation while ensuring patient safety and ethical AI development.
The Future of AI in Biologics: Balancing Promise and Responsibility
The future of AI in biologics drug discovery holds immense promise, but realizing its full potential requires careful navigation of both opportunities and challenges. The convergence of AI, big data, and advanced biological research is poised to transform the pharmaceutical industry, potentially leading to a new era of personalized medicine and faster development of life-saving therapies. However, the successful integration of AI hinges on addressing ethical considerations, developing robust regulatory frameworks, and fostering collaboration among stakeholders. By prioritizing data quality, promoting transparency and explainability in AI algorithms, and ensuring equitable access to the benefits of AI-driven healthcare, we can unlock the transformative power of AI to improve human health while mitigating potential risks. The journey ahead requires a collective commitment to responsible AI development and a shared vision for a future where AI empowers us to conquer disease and enhance human well-being.
----------
Further Reads
I. https://pmc.ncbi.nlm.nih.gov/articles/PMC7577280/
II. https://pmc.ncbi.nlm.nih.gov/articles/PMC10302550/
III. https://www.biopharmatrend.com/post/590-10-companies-applying-ai-for-biologics-discovery/