Key Takeaways:
I. Command R7B's efficiency and speed challenge the assumption that model size equates to performance.
II. Multilingual support in Command R7B opens doors to global accessibility but requires careful consideration of data bias and evaluation challenges.
III. Command R7B's cost-effectiveness and accessibility have the potential to disrupt the enterprise AI market, empowering smaller businesses and driving innovation.
In the rapidly evolving landscape of enterprise AI, the demand for efficient and accessible language models is intensifying. Cohere, a leading AI startup, has responded to this demand with the release of Command R7B, the smallest and fastest model in its R series. Designed for rapid prototyping and iteration, Command R7B leverages Retrieval Augmented Generation (RAG) to enhance accuracy and boasts a 128K context length, supporting an impressive 23 languages. This launch signals a potential shift towards more efficient and accessible RAG solutions, challenging the prevailing notion that larger models are inherently superior. This article delves into the technical advancements, performance claims, and strategic implications of Command R7B, providing a nuanced perspective on its potential to reshape the enterprise AI landscape.
Performance Benchmarking: A Critical Assessment
Command R7B, with its 7 billion parameters, is significantly smaller than many leading large language models (LLMs). This smaller size translates to faster inference speeds and reduced computational requirements, making it a potentially attractive option for resource-constrained environments. Cohere claims that despite its smaller size, Command R7B outperforms competitors like Google's Gemma, Meta's Llama, and Mistral's Ministral in tasks such as math, coding, and retrieval augmented generation (RAG). These claims, however, are not fully substantiated by publicly available benchmark data, making independent verification crucial for a thorough assessment.
While Cohere reports strong performance on internal benchmarks and highlights its top ranking in areas like instruction-following evaluation (IFeval), big bench hard (BBH), and graduate-level Google-proof Q&A (GPQA), the absence of readily accessible, standardized benchmark results makes it challenging to compare Command R7B directly against its competitors. Transparency and independent validation are essential for building trust and allowing for a fair assessment of the model's true capabilities. The AI research community requires access to comprehensive benchmark data to evaluate the trade-offs between model size, speed, and accuracy.
From a technical perspective, Command R7B incorporates several architectural innovations that contribute to its efficiency. The use of a sliding window attention mechanism with a window size of 4096, combined with Rotary Position Embedding (ROPE), allows the model to handle long context lengths efficiently. The inclusion of a fourth layer with global attention further enhances its ability to capture complex relationships within the text. These technical details, while promising, require further investigation through independent benchmarking to determine their real-world impact on performance.
Ultimately, the value of any LLM lies in its practical applicability and demonstrable performance on real-world tasks. While smaller size and faster speeds are attractive features, they should not come at the expense of accuracy and robustness. The lack of comprehensive benchmark data for Command R7B necessitates a cautious approach to evaluating its true potential. Further research and independent validation are essential to determine whether it truly delivers on its promise of superior performance while maintaining the accuracy required for demanding enterprise applications.
23 Languages and Counting: The Challenges and Opportunities of Multilingual AI
Command R7B's support for 23 languages is a significant step towards making AI more accessible and inclusive on a global scale. This multilingual capability allows businesses and developers to build applications that cater to a diverse range of users, opening up new markets and opportunities for cross-cultural communication and collaboration. This broad language support is particularly valuable in today's interconnected world, where language barriers can hinder innovation and limit access to information and technology.
Developing and deploying effective multilingual models presents significant technical challenges. Data scarcity for some languages can lead to performance disparities, while biases present in training data can perpetuate and amplify existing societal inequalities. Ensuring equitable performance across all supported languages requires careful data curation, advanced techniques like cross-lingual transfer learning, and ongoing efforts to mitigate bias and promote fairness.
Cohere's approach to multilingual training involves utilizing a massive multilingual corpus and employing techniques like cross-lingual transfer learning. This approach aims to leverage knowledge gained from high-resource languages to improve performance in low-resource languages, striving for a more balanced and equitable outcome. However, the effectiveness of these techniques in mitigating bias and ensuring consistent performance across all 23 languages requires further investigation and transparent evaluation.
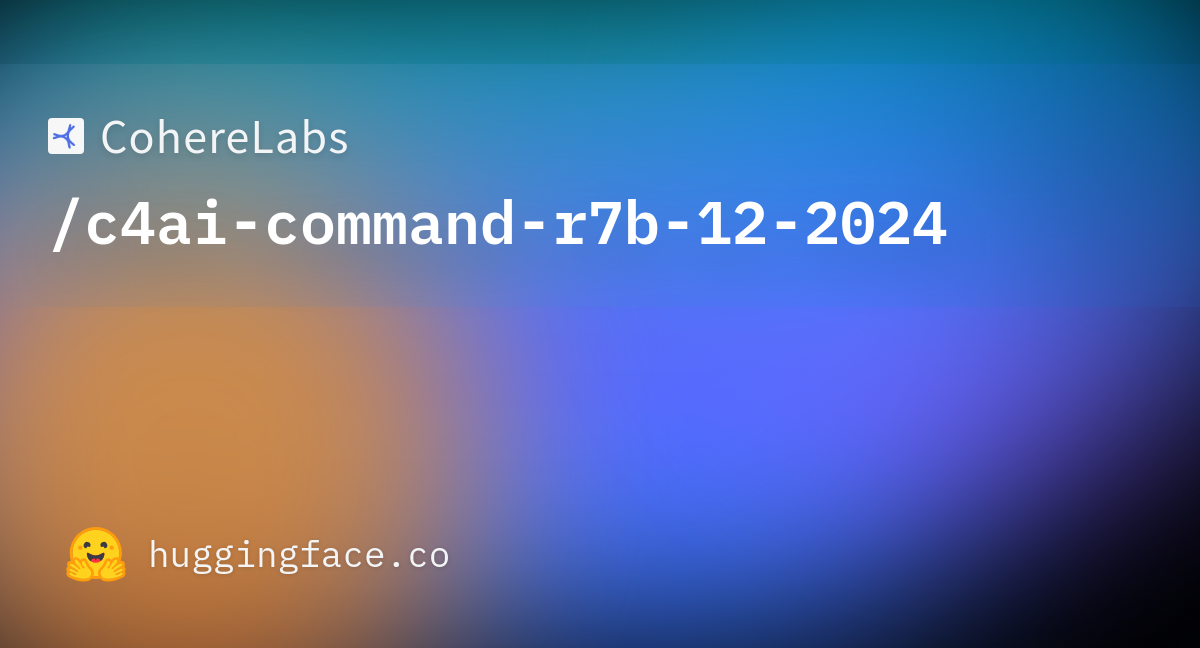
The true measure of success for multilingual AI models lies not just in the number of languages supported, but in their ability to perform equitably and ethically across diverse linguistic landscapes. Addressing potential biases, ensuring fairness, and promoting inclusivity are paramount for building AI systems that truly bridge linguistic divides and empower individuals and communities worldwide. Continuous research, development, and open dialogue are essential for navigating the complexities of multilingual AI and realizing its full potential.
Cost-Effectiveness and Market Disruption
Command R7B's smaller size and optimized architecture translate to significant cost advantages compared to larger, more resource-intensive models. Its ability to run efficiently on commodity hardware, including MacBooks, reduces the barrier to entry for smaller businesses and individual developers, potentially democratizing access to advanced AI capabilities. This cost-effectiveness could disrupt the enterprise AI market, challenging the dominance of resource-intensive solutions and empowering a wider range of players. The pricing model, set at $0.0375 per 1 million input tokens and $0.15 per 1 million output tokens, further enhances its accessibility.
The introduction of a cost-effective and efficient model like Command R7B has the potential to reshape the competitive landscape of the enterprise AI market. Existing players offering larger, more resource-intensive solutions may face pressure to adapt their pricing and offerings. Smaller companies could gain a competitive edge by leveraging Command R7B's accessibility to develop innovative AI-powered products and services. The long-term impact on market dynamics will depend on factors such as the model's real-world performance, the evolution of the competitive landscape, and the adoption rate by businesses of all sizes.
Command R7B and the Evolving AI Landscape
Cohere's Command R7B represents a notable development in the ongoing evolution of enterprise AI. Its focus on efficiency, accessibility, and multilingual capabilities challenges the prevailing narrative that bigger is always better. While the model's claimed performance advantages require further validation through independent, standardized benchmarks, its potential to democratize access to advanced AI is undeniable. The long-term success of Command R7B, and indeed the broader trend towards efficient AI, will depend on striking a balance between performance, accessibility, and responsible development. As the AI landscape continues to evolve, a nuanced and critical approach to evaluating new technologies will be essential for navigating the complexities and realizing the full potential of AI for businesses and society as a whole.
----------
Further Reads
I. The Command R Model — Cohere
II. CohereForAI/c4ai-command-r7b-12-2024 · Hugging Face
III. RAG-Instruct Capabilities: “They Grow up So Fast” | by Darren Oberst | Medium